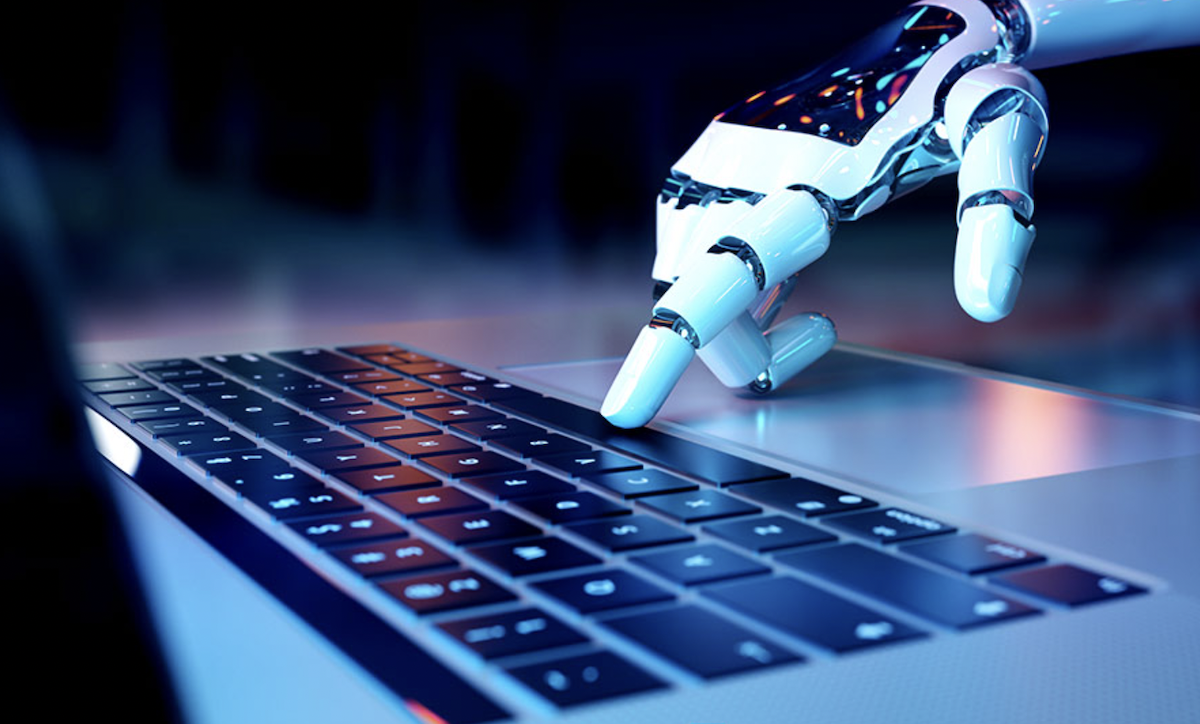
The breadth of its application can explain the great demand for technology around the world. For example, machine learning solutions work in advertising, financial, IT, as well as in IT security and process automation.
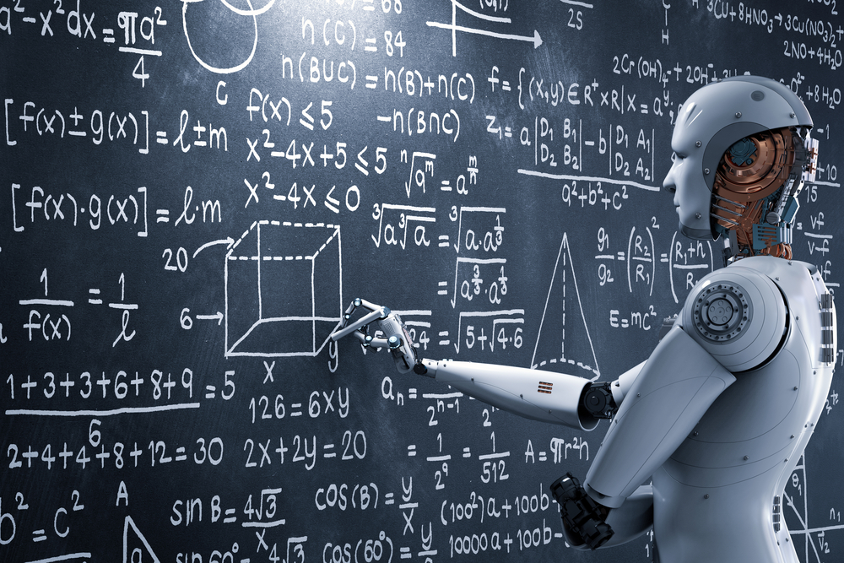
Table of Contents
What Kinds of Business Problems Can Machine Learning Handle
Machine learning is important when a company has a lot of data and needs to simplify working with them or earn more with their help. Such a scenario is relevant, for example, for marketing, IT and banking spheres.
Personalization of user interaction
Automation and online work has led to the fact that businesses have accumulated large amounts of data. This is not only information about each client. It also about data on demand, seasonality, user preferences and their behavior on the network. Machine learning is used to turn this data into hypotheses, based on which you can personalize your work with users.
Forecasting
Based on the collected data, algorithms can predict the future. An increase in demand, seasonality or customer outflow. Adobe, which has learned to analyze user experience and work with dissatisfied customers in automatic mode. It solves for example, thanks to machine learning.
Internet of Things
The growing popularity of smart homes and gadgets will lead to the fact that companies will accumulate users’ information. Based on them, scenarios and patterns will be developed that will improve the user experience.
IT security
For example, the list of top proxies offers comfortable proxies that can be used in many business areas. It also used this approach in the search for various “anomalies” in user actions. For example, MasterCard analyzes transactions, purchase data, geolocation and other information. In order to combat fraudulent actions and protect money from users’ bank cards.
Guidelines for applying machine learning to business tasks
As before, your favorite goal, you need to make a plan and structure for implementing machine learning
The goal
We need to start by defining the goal that we want to achieve. This can be an increase in sales, an increase in the average check, cost reduction, reduction of errors or acceleration of processes. It is important that the parameters are measurable and achievable. For example, revenue growth by at least 30%, conversion increase by 45%.
Processes related to cognitive services are most often automated through smart video surveillance. This allows you to reduce the percentage of defects in finished products. Also increase safety and automate several processes.
Creating a culture of working with data
Any process related to artificial intelligence requires a large amount of data. Over the past 10 years, we have accumulated a large array of data such as texts, photos, video images, and records in CRM and ERP.
Data collection and storage requires a certain culture of working with data. Each employee should understand all incoming and outgoing information for further analysis.
Data collection and processing
Data collection may take some time. The word “big” in the term Big Data is not accidental. The data should level statistical errors, training models, forming a control sample to determine the quality of models.
Building models
When the preparatory work is completed, it builds ensembles of models. They are trained on pre-collected data (usually 75% of the total sample), then the resulting models are tested on a control sample (the remaining 25%). Those models whose prediction coincided with the real data more accurately are suitable.
Of course, this description is significantly simplified. In reality, the breakdown into test and training samples is mixed several times. It is needed to improve the accuracy of the model and eliminate accidental coincidence of the results.
Checking results and brainstorms
When the models are built, the test data is predicted accurately, it is necessary to check the results on the combat data. This is not a straightforward task. Since it is important to neutralize various cognitive distortions caused by the very presence of the forecast. The verification of models should be carried out considering the leveling of these difficulties.
Business analysts will receive information about users. It will allow them to better understand their audience for improving the sales.
Refinement of models
The information obtained because of checking on real up-to-date data. Even if it significantly contradicts the results of forecasts, it will help refine the model. It may relate discrepancies to changes in the data itself (archived data may differ from current ones), or to the problem described above. The constructed model is tested on a new array of data and enriched with them.
Final Thoughts
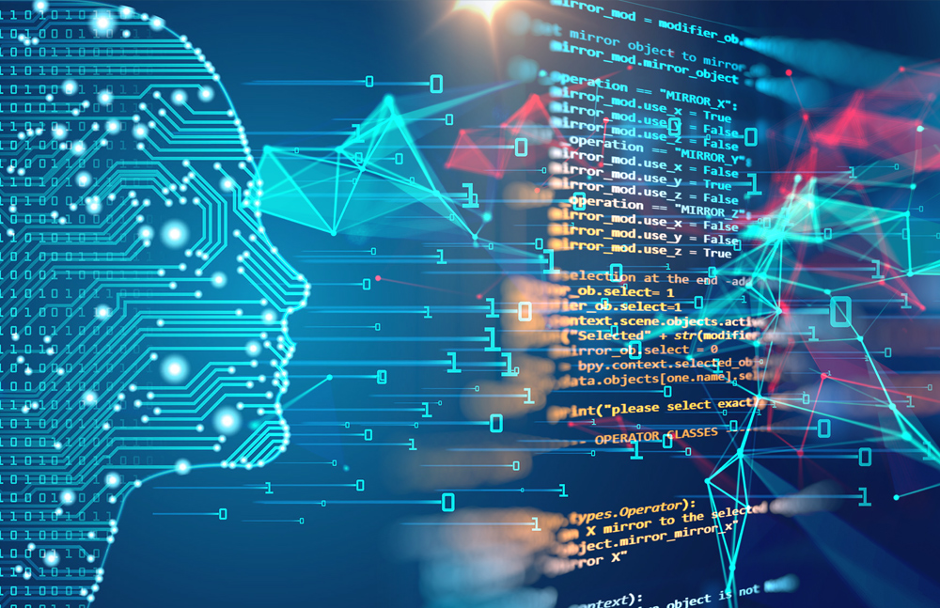
Although machine learning has existed for a long time, the technology has been actively developing only in the last few years. Usually this is not enough to guess the future of the sphere. However, experts’ forecasts suggest that the popularity of machine learning will grow as the volume of user data increases.
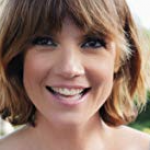