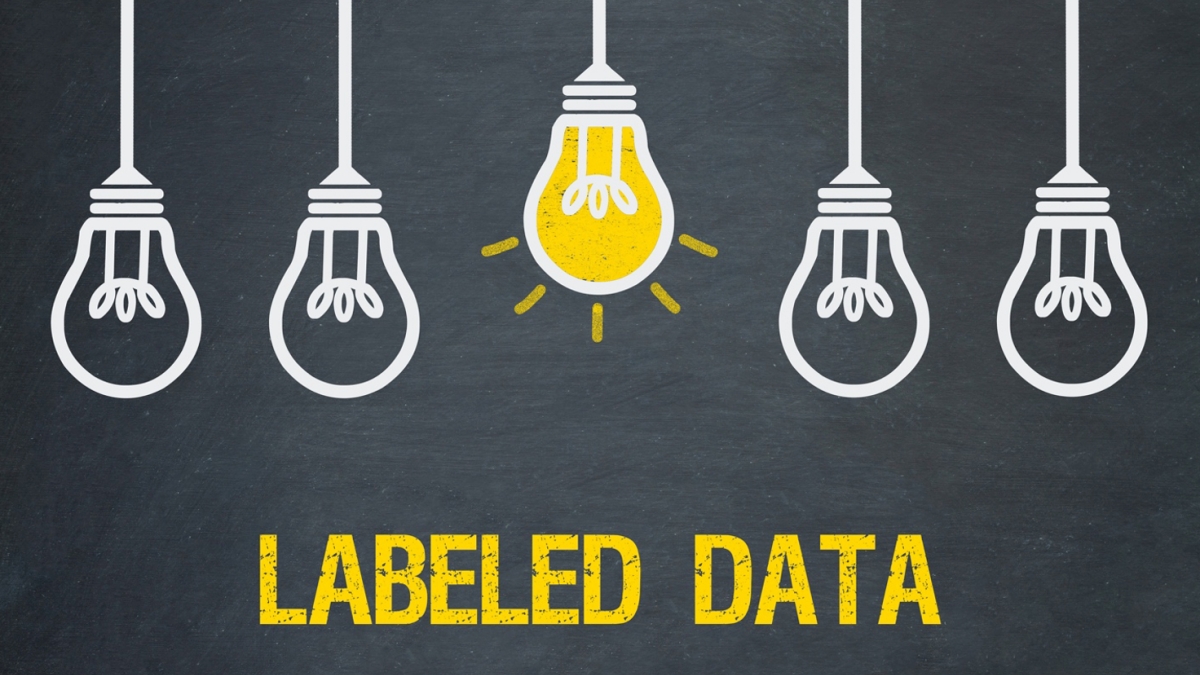
The modern world runs on digitized data. Everything you do on your devices relies on it. Even the written code that comprises all software is considered data. Different types of data are at the core of their functionality in advanced software like Artificial Intelligence (AI) and Machine Learning (ML), which many people have heard of.
In 2021, 86% of CEOs reported using AI for business. Even smartphones employ AI to achieve voice recognition. With how widespread these technologies are becoming, it’s easy to disregard the underlying complexities that make them possible. Thus, a data labelling platform is a software that allows these computerized systems to mimic human behavior and learn. This article will explain data labeling and some of its various applications. (1)
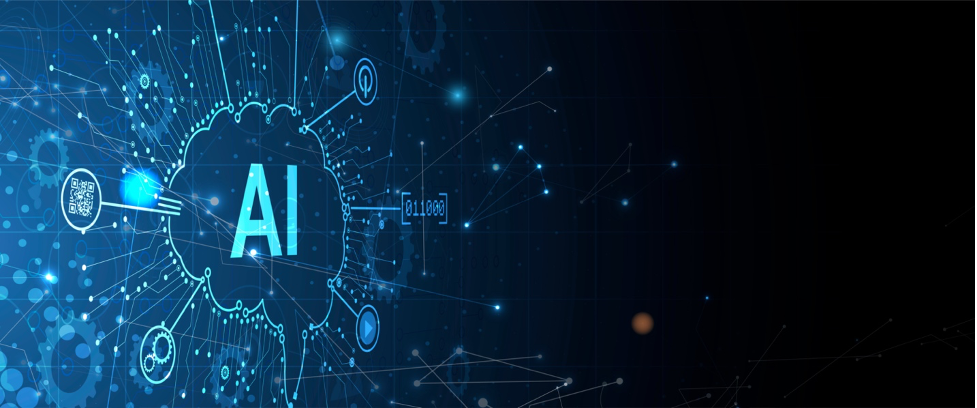
Table of Contents
Understanding Data Labeling
First of all, it’s essential to understand that data can be any type of information stored on a computer. Data labeling is adding different “labels” or “tags” to various pieces of data to be used in ML or any other type of AI.
To simplify, data with a label is “labeled data” while data without it is “unlabeled data.” As you can imagine, far more can be done with the former than with the latter. Machines are meant to process data; hence, they tend to misinterpret when they aren’t guided. While it might seem simple, a computer can’t automatically understand what data it’s given without being told. These “labels” are how machines understand data and process it appropriately.
Imagine a dataset containing two images and a computer system that sorts images of animals by type. One of these images is of a dog, while the other is of a cat. These two images are currently unlabeled data. The computer system knows it’s got two images but doesn’t know what to do with them. Give these images a label saying “dog” and “cat,” respectively, and the system will then be able to understand what the images contain and sort them properly.
The Applications of Data Labeling
Machine learning can be split into two broad categories: unsupervised and supervised learning. The distinction here is that supervised learning uses labeled data, and unsupervised learning uses unlabeled data. On average, 80% of the time spent on a supervised learning project involves labeling data. Looking at the applications of data labeling means looking at supervised machine learning applications. (2)
Labeled data can for example be leveraged by Neural networks , which is a branch of supervised machine learning. This field of study involves creating ML systems that is inpired by how the human brain learns. Some of its applications include defense, facial recognition, handwriting analysis, and healthcare.
Here are some of the most prominent current applications of data labeling:
. Audio Recognition
Speech-to-text software and voice commands are great examples of this that you might have already encountered. Audio recognition involves analyzing sound and converting it to a labeled data set used by ML software.
These ML computer systems take various sounds and convert them to data that the system is capable of understanding. For example, a voice command software identifies your voice, converts it to information that it can recognize, and compares it to its pre-existing data to determine what you’re saying.
. Text Processing
Language translation, text auto-completion, and spelling/grammar checking apps use text processing. Different words, text, and symbols are labeled. These pieces of labeled data are recognized by text processing ML software. For example, a language translation app might label a word as a particular translation and a particular part of speech. When you give the software a sentence to translate, it uses these labels to identify each word and provide an appropriate translation.i.Social Media and Digital Marketing
You’re probably already familiar with at least one social media platform. Think about how it works. People can add tags to their posts, which effectively means that these posts become “labeled data.”
Most social media platforms use an ML or AI algorithm at their core. These algorithms use tags on posts to understand and categorize the content within the post. This allows social media apps to learn what sort of content a user enjoys and suggest the user content they might enjoy. Similarly, this is how social media engines can show targeted advertisements to users who are likely to be interested.
Conclusion
Data labeling is the process of giving “labels” or “tags” to different pieces of information to be understood by artificial intelligence and machine learning systems. The type of machine learning that uses labeled data is called “supervised learning.” Its applications are pretty vast. Moreover, they can be used for almost anything, from marketing to identifying early-stage diseases.
Using data labeling can help you drastically improve your artificial intelligence projects, life, or business. This technology is still growing as machine learning grows. If it’s capable of so much, imagine what it’ll be able to do in the future.
References:
- “65 Artificial Intelligence Statistics for 2021 and Beyond”, Source: https://www.semrush.com/blog/artificial-intelligence-stats/
- “What is Data Labeling? Everything You Need To Know With Meeta Dash”, Source: https://appen.com/blog/data-labeling/
- “Frontiers | Identifying Disease Related Genes by Network Representation and Convolutional Neural Network | Cell and Developmental Biology”, Source: https://www.frontiersin.org/articles/10.3389/fcell.2021.629876/full
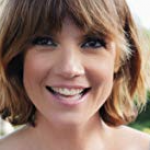