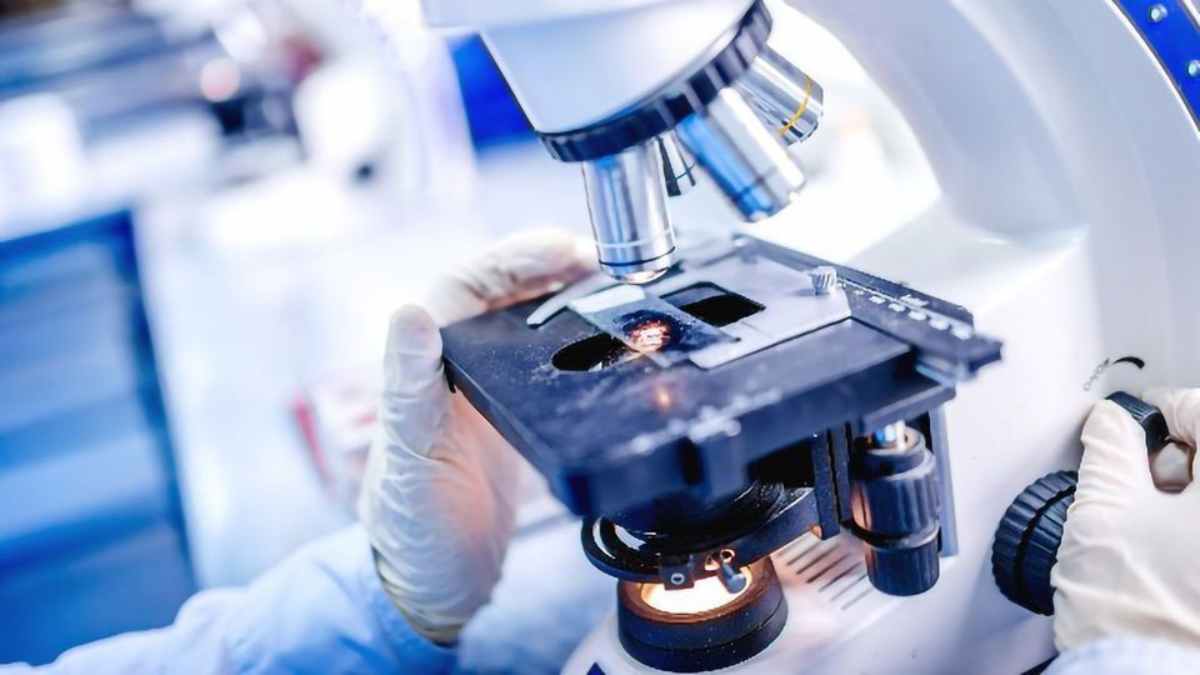
Microarray reagents are commonly used in gene expression analysis, particularly for analyzing RNA expression. This technology offers a high-throughput approach to studying gene expression patterns, allowing researchers to study multiple genes simultaneously. However, there are certain best practices that must be followed when using microarray reagents for RNA expression analysis, as well as common pitfalls that should be avoided.
Table of Contents
Importance of RNA Expression Analysis
RNA expression analysis plays a crucial role in many areas of research, including genetics, molecular biology, and medicine. By measuring the amount of RNA produced by specific genes in different tissues or under different experimental conditions, researchers can gain insight into the biological processes involved in disease development, drug response, and other phenomena. Microarray reagents are often used in this type of analysis because they allow for the measurement of hundreds or even thousands of genes at once.
Best Practices for Analyzing RNA Expression with Microarray Reagents
To ensure accurate and reliable results when using microarray reagents for RNA expression analysis, it is important to follow certain best practices. These include:
Sample Preparation:
High-quality samples are essential for accurate gene expression analysis. The first step is to extract RNA from the sample tissue or cells using a method that minimizes degradation and contamination. Once extracted, the quality and quantity of the RNA should be assessed using spectrophotometry or electrophoresis.
Labeling and Hybridization:
The next step is to label the extracted RNA with fluorescent dyes before hybridizing it to the microarray slide. Choosing an appropriate labeling method is critical as it can affect sensitivity and specificity. Hybridization optimization is also important since hybridization efficiency can vary depending on factors such as temperature and buffer composition.
Microarray Scanning and Data Acquisition:
After hybridization is complete, the microarray slide needs to be scanned using a high-resolution scanner capable of detecting fluorescence signals from each spot on the slide. Images obtained from scanning need normalization before data interpretation.
Common Pitfalls To Avoid When Using Microarray Reagents For RNA Expression Analysis
Despite its many advantages, there are several common pitfalls associated with using microarray reagents for RNA expression analysis that should be avoided:
Sample Handling Errors:
One of the common pitfalls to avoid when using microarray reagents for RNA expression analysis is sample handling errors. Samples can be degraded if not handled properly during extraction or storage, leading to inaccurate results. RNA is a highly unstable molecule that can easily degrade in response to environmental factors such as heat, light, and oxidizing agents. Therefore, it is essential to take proper precautions during sample collection, storage, and processing to minimize the risk of degradation. For example, samples should be stored at -80°C immediately after extraction and thawed only once before use. Any deviations from these standard procedures can have a significant impact on gene expression data interpretation and lead to erroneous conclusions being drawn from the data analyses.
Poor Labeling Efficiency Or Accuracy:
Another common pitfall to avoid when using microarray reagents for RNA expression analysis is poor labeling efficiency or accuracy. Poor labeling can lead to low signal-to-noise ratio, resulting in inaccurate results. Since microarray technology relies on fluorescent signals emitted by the labeled RNA molecules, any errors in labeling can significantly affect the sensitivity and specificity of gene expression measurements. Therefore, it is crucial to choose an appropriate labeling method that ensures high efficiency and accuracy while minimizing variability between samples. Additionally, quality control measures should be implemented at each step of the labeling process to ensure that only high-quality samples are used for downstream analysis. By following these best practices, researchers can minimize the risk of poor labeling and obtain more accurate and reliable results from their microarray-based RNA expression studies.
Incomplete Or Improper Data Normalization:
Incomplete or improper data normalization is another common pitfall that can lead to false positives/negatives and wrong conclusions being drawn from data analyses. Normalization is a critical step in microarray data analysis that involves adjusting raw gene expression values to account for technical variation between samples. If normalization is incomplete or performed improperly, it can result in biased estimates of gene expression levels, leading to erroneous conclusions. Therefore, it is essential to choose an appropriate normalization method based on the characteristics of the data and perform quality control checks to ensure that only high-quality normalized data are used for downstream analysis. By implementing best practices in data normalization, researchers can minimize the risk of false positives/negatives and obtain more accurate and reliable results from their microarray-based RNA expression studies.
Conclusion
In conclusion, while microarray reagents offer a high-throughput approach for analyzing gene expression patterns accurately; best practices must be followed while avoiding common pitfalls that could compromise result integrity. Careful consideration should be given throughout all steps in this process – sample preparation through data normalization – ensuring accurate conclusions may ultimately emerge from these studies.
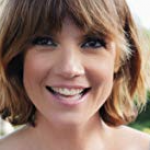