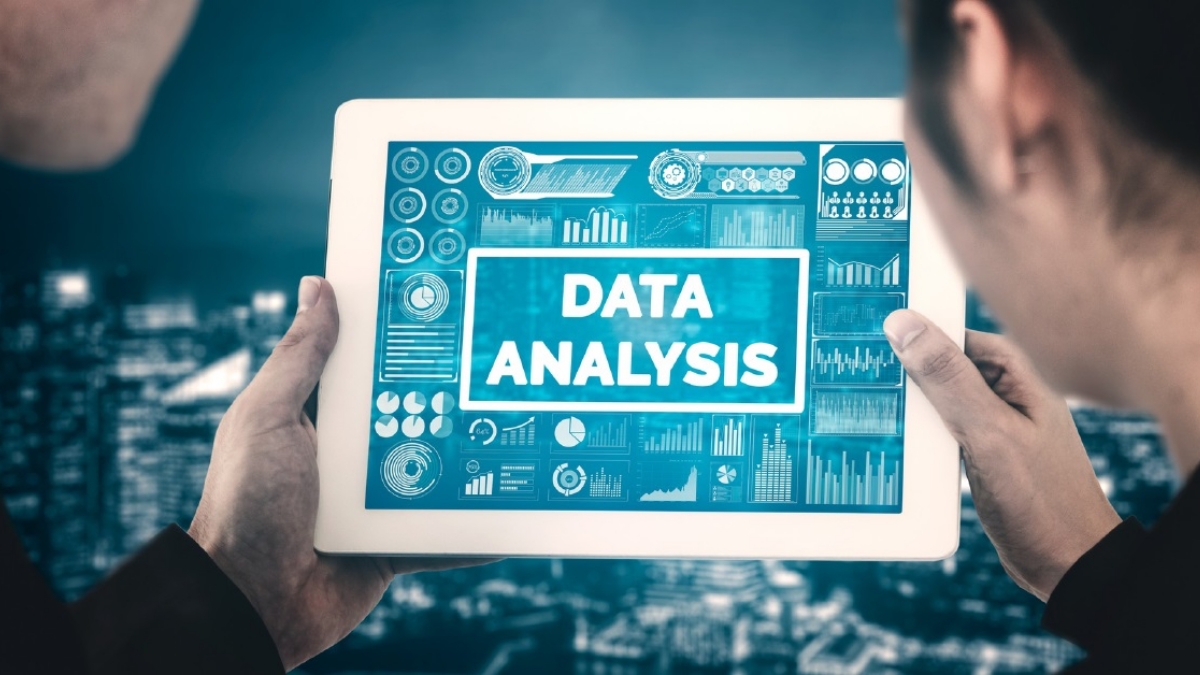
In today’s rapidly evolving digital landscape, businesses are inundated with overwhelming volumes of data. Yet, a significant portion of this data is unstructured, residing in forms like customer reviews, social media posts and feedback. Extracting meaningful insights from such diverse data sources can be challenging. This is where Natural Language Processing (NLP) comes into play, revolutionizing how businesses interpret and utilize data. With the help of platforms like Kyvos, companies can seamlessly integrate NLP into their analytics processes, unleashing a new era of data-driven decision-making.
Table of Contents
Understanding Natural Language Processing and Its Impact
Natural Language Processing (NLP) is a branch of artificial intelligence that focuses on the interaction between computers and humans through natural language. It enables machines to understand, interpret and respond to human languages in a valuable way. This capability is transforming how businesses handle data analytics. With NLP, companies can quickly sift through colossal amounts of text data, identify patterns and extract actionable insights.
Why NLP is Essential for Modern Data Analytics
In a world teeming with data, the ability to extract insights from unstructured sources is not just an advantage—it’s a necessity. NLP enables businesses to unlock the potential of text data, transforming it into valuable insights. Whether it’s analyzing customer feedback, monitoring brand sentiment on social media, or interpreting product reviews, NLP offers unparalleled insight.
Real-world examples showcase NLP’s profound impact across various sectors. In retail, companies use NLP to analyze customer reviews and fine-tune their offerings. Healthcare organizations utilize NLP to extract critical information from medical records, enhancing patient care. Meanwhile, in finance, NLP helps institutions assess risks and trends by analyzing market reports and news articles. These applications underscore NLP’s critical role in enhancing decision-making and driving business success.
Navigating Challenges in Traditional Data Analytics
Despite the promise of data analytics, traditional tools often falter when faced with unstructured text data. Conventional analytics solutions struggle to process vast volumes of text, leading to inefficiencies and missed opportunities. Data silos, where information is trapped within separate systems, further hinder the analytics process. Additionally, the lack of context in traditional approaches can result in misleading conclusions.
Slow processing times are another common issue in traditional analytics setups. Without the power of NLP, organizations face delays in extracting insights, impacting their agility and responsiveness. These challenges highlight the need for advanced solutions that can bridge the gap between raw data and actionable insights, enabling businesses to stay competitive.
Integrating NLP with Advanced Data Analytics Platforms
Businesses can overcome the limitations of traditional analytics tools by integrating NLP with platforms that offer real-time analytics, intelligent data aggregation for instant insights and advanced search capabilities. These platforms simplify the process of running NLP models at scale, addressing common challenges like data governance and latency. They enable companies to access insights in the moment, rather than waiting for batch processing. This capability is particularly valuable in fast-paced industries where timely decisions are paramount.
With NLP, businesses can perform complex queries and receive precise results, all while maintaining data integrity.
Applications of NLP for Advanced Data Analytics
NLP has a diverse range of applications that impact multiple areas of business. Some key examples include:
- Customer sentiment analysis: NLP can help businesses analyze customer feedback and reviews to identify trends, preferences and sentiments towards products or services. For instance, NLP uses supervised machine learning models, trained on large datasets of labeled sentiment data (e.g., positive, negative, or neutral reviews). These models can automatically classify new customer feedback based on patterns they’ve learned.
- Social media monitoring: By analyzing social media posts, NLP can help businesses track brand sentiment, monitor online conversations and understand customer behavior. For example, NLP can identify the most frequently mentioned words or hashtags related to the brand or product. This helps in discovering trending topics.
- Insight Extraction from Text Data: NLP can sift through vast amounts of unstructured data to extract valuable insights for decision-making. It breaks down customer feedback into individual words or phrases (called tokens). This makes the text easier to analyze. Unnecessary elements like punctuation, stop words (e.g., “the,” “is,” etc.) and irrelevant data are removed to focus on meaningful words. Then techniques like stemming or lemmatization are applied to reduce words to their base form (e.g., “running” becomes “run”). This helps in grouping similar words.
- Risk Assessment: In finance, NLP can assist in evaluating market risks by analyzing news articles and reports in real-time. For example, a financial institution could use NLP to scan thousands of news sources for mentions of terms like “economic downturn” or “regulatory changes.” If a report highlights potential risks in a particular industry or geopolitical tensions, NLP algorithms can flag this information immediately, allowing the institution to adjust its investment strategies or hedge against potential losses. This helps businesses make faster, more informed decisions to mitigate financial risks.
- Language Translation: With the help of NLP, businesses can translate text data from one language to another in real-time, aiding communication and decision-making in a global context. It enables real-time translation by leveraging advanced machine learning models like neural machine translation (NMT). These models are trained on vast datasets of multilingual text to understand the structure, grammar and context of different languages. When a business needs to translate text data, NLP processes the input by identifying key words, phrases and their meanings in the source language, then converts it into the target language while preserving context and meaning. This real-time translation helps businesses overcome language barriers, facilitating global communication and aiding faster decision-making in international markets.
Conclusion
NLP is revolutionizing how companies handle data analytics. By seamlessly integrating NLP with advanced analytics platforms, businesses can unlock the full potential of unstructured text data. With its wide range of applications and its ability to provide valuable insights, NLP is an essential tool for modern data-driven decision-making. As the volume and complexity of data continue to increase, leveraging NLP will be crucial for staying competitive in today’s digital landscape.
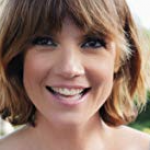