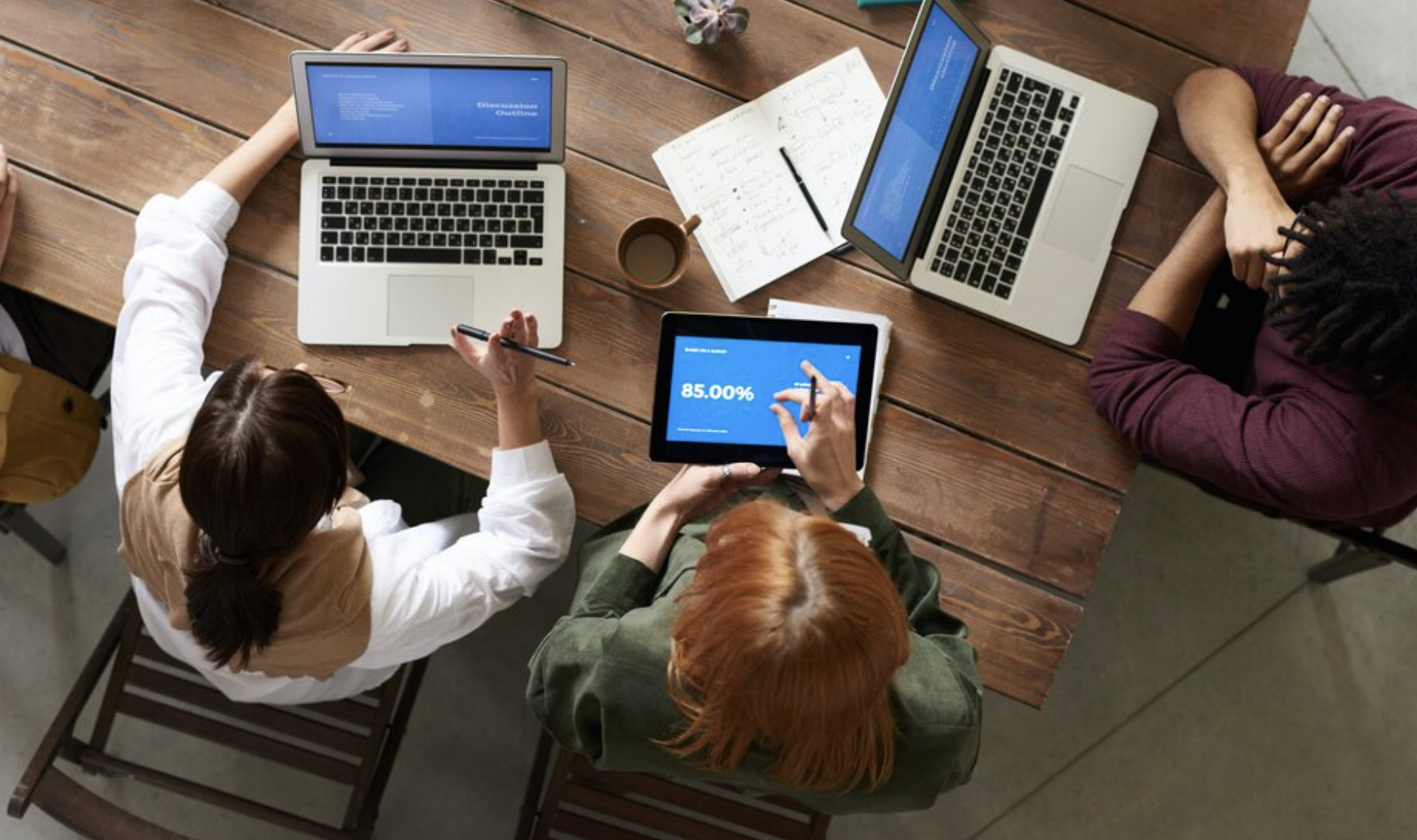
Companies crave to use relevant data and information to expand and improve their performance, but enterprises are struggling with searching now. Knowledge is core to business insights, and there are strong costs and risks connected with the time spent searching for information or erroneous decisions based on fallacious information.
Table of Contents
How Intelligent Searching Can Help
Intelligent searching—powered by machine learning—helps resolve these challenges by utilizing natural language intelligence and deep learning to distinguish areas for organizational transformation, including the following:
- Expediting analysis and growth
- Enhancing customer self-service and consumer content accounts
- Lessening governing compliance expenses
Questions To Ask Before Plunging Into Artificial Intelligent Searching
Before engaging in the hopes and opportunities of artificial intelligence (AI), organizations need to take a step backward and comprehend the following:
- Can intelligent searching be ‘smart’ enough to provide the relevant results we need?
- If we deploy AI tools (like a chatbot), can we eliminate the need for a well-defined searching capability?
- Will artificial intelligence naturally ‘happen’ without any content optimization or taxonomy processes?
These questions are occurring more frequently as the promises of AI become further powerful. Unfortunately, some market vendors are not administering this accessibility as they communicate their tool’s capabilities as the best and smartest. So what exactly is the truth ahead of the hype?
The Reality Behind ‘Intelligent Searching’
Whenever enterprises want to introduce, say a chatbot, instead of investing in intelligent searching, they need to confirm the following in the first instance:
- How will we know that the results are ‘relevant’ if we do not completely understand the quality of our content?
- How will we comprehend the answers that the AI chatbot presents are really what our customers and end-users need?
- How do we know if or when the ‘intelligent’ searching process is sufficiently intelligent?
- How can we measure its success (and hence understand our return on investment (ROI)) if we do not understand how it operates because it’s basically an ‘intelligent’ black box?
- Can an ‘intelligent’ searching tool be adequately intelligent to supplant human content compilation?
Do Not Expect Incantation
What this all implies in practice is that enterprises must be smart enough and do the required due diligence and planning to accommodate these ‘intelligent’ searching tools so they can help their end users later. So what does ‘help’ resemble in this circumstance? To help the tool better help yourself later on, enterprises will need robust taxonomies, training use case sets, and a deep understanding of their content, end users, and requirements before injecting such a tool.
This is not to assume that these emerging technologies are cumbersome and time-consuming for initial deployment and continuous improvement. On the contrary, they are more than helpful in numerous organizations, but only if deployed and managed with the right approach. Enterprises should not expect instant magic because even these intelligent tools need a specific level of initial ‘manhandling’—and you do not want them to surprise you unnecessarily later on.
Enterprises yearning to embrace intelligent searching need to internally perpetrate by leveraging their statistical business data and utilizing functional AI technologies to completely recognize their end-user search intent. This technical approach is needed to achieve the AI-motivated, intelligent searching experience that end users will both expect and demand.
Natural language processing (NLP) tools both create and cultivate a customized dictionary for enterprises, which encourages the search application to learn the language presented within searching queries and searchable documents. Recorded end-user query and click log files can be electronically ‘mined,’ and statistical analysis will systematically measure and advance the searching relevance as time moves forward.
Machine learning and NLP modeling automatically extract and synthesize all pertinent information from the searchable content. This process provides an alternative to rigidly rule-based approaches, launching extraction and classification processes that are further scalable and efficient when studying large quantities of data content.
These ‘training’ (or innovative work-in-process) intelligent searching models will demand an appropriate and consistent data set of all searchable content. Accordingly, growing these data sets serves as a single key challenge for any enterprise embarking on the implementation process.
The relevant information extricated from intelligent searchable content can populate knowledge databases, presenting improved searching performances—resulting in more than suitable searching solutions.
Conclusion
Aligning intelligent searching technologies with an enterprise’s both current and future technology needs is a natural senior management directive. Enterprises will endeavor to increase the ROI of their intelligent searching investment, and the smart searching maturity process will eventually provide reasonable guidelines for maximizing deployment achievement.
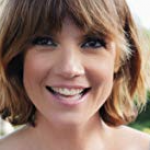